Deprofessionalization
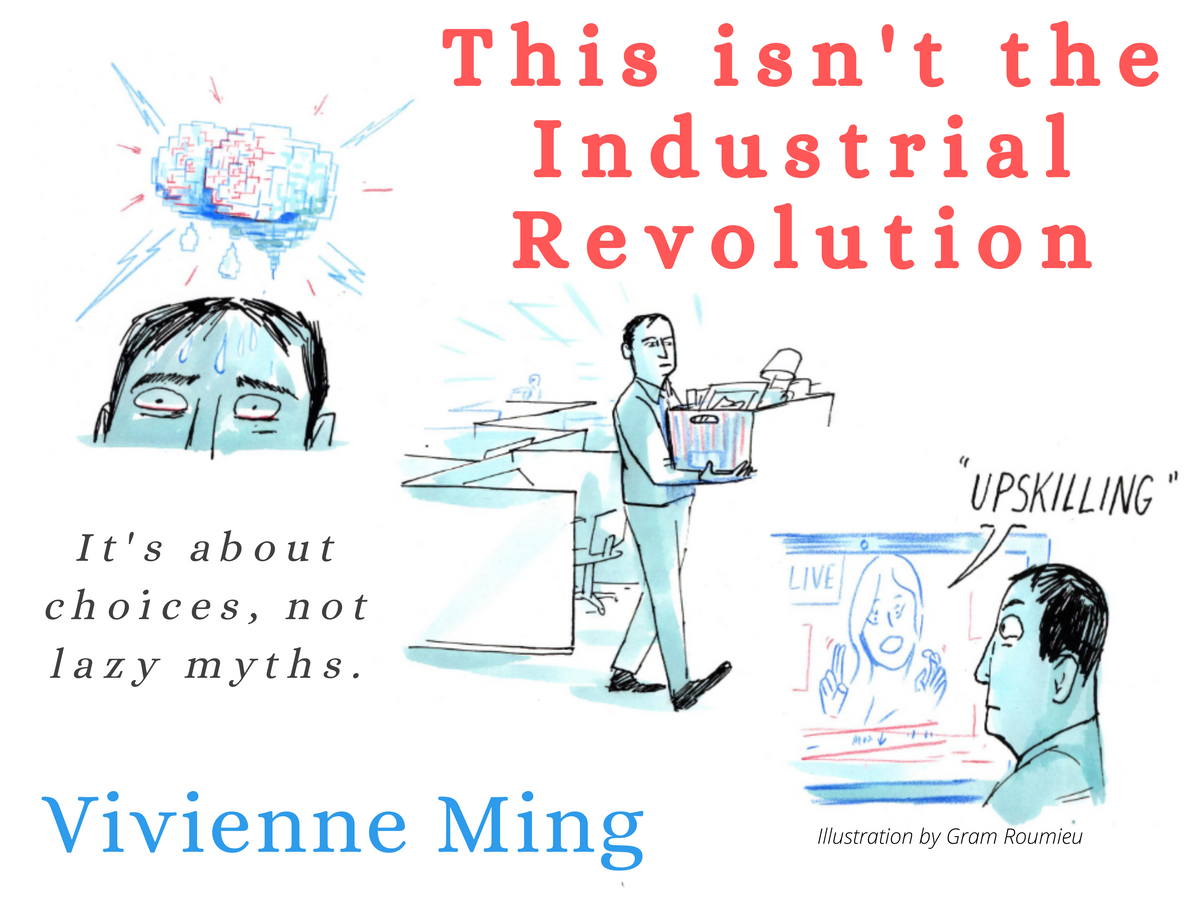
Deprofessionalization
“AI will create superdoctors by taking away all of the busywork and leaving only the fun stuff...We don’t even need universities anymore; we can just send them all to trade school to get upskilled.”
– Pompous Mansplainer
“I don’t know why you’re so worried about it. We’re already teaching coal miners how to program. And social skills! AI can’t do social, so we can train everyone to be elder care workers.”
– Pompous Mansplainer
Automating Diabetes40
On Thanksgiving day of 2012, my son was diagnosed with Type-1 diabetes. As I have shared many times before, my son and I spent the long holiday weekend at Oakland Children’s Hospital, surrounded by bright lights, beeping machines, medical staff, and hospital cafeteria turkey. I’ll skip the details here; suffice to say I do not recommend this Airbnb host41. After a few excruciating days of titrating his blood glucose level down to a survivable range, he was out of the woods, and my wife and I were faced with a whole new parenting responsibility: managing our son’s chronic, life-threatening illness.
We immediately read all of the research we could find on diabetes and soon began collecting as much data from our son as possible. This included data on blood glucose levels, heart rate, sniffles, grumpiness, activity levels, nutrition, and everything else we could quantify. In an astonishing display of naivete, we presented our work to his doctor in our first out-patient visit post-diagnosis (thousands of data points collected daily covering reams and reams of paper). The staff was not happy. “How dare you waste our time with this. What are we supposed to do with all of this data?” They responded by handing us a photocopied sheet of paper with instructions to write down 15 blood glucose readings: morning, afternoon, and night for 5 days. They eyeballed those 15 numbers rather than our 15 thousand to design the treatment plan for the next three months of our son’s life.
These doctors and nurses were all highly educated. They had seen literally thousands more cases of type-1 diabetes than I had and possessed an expertise my fevered research reading would never equal. However, when offered an incredible but novel resource–scientist parents providing sophisticated data and willing to explore complex treatments–these doctors chose their daily routine, down to the at-a-glance numbers they reviewed. They had an established process for treating diabetes, and a whole lot of patients in their long days. Exploring some crazy new method for treating one kid struck them as an absurd waste of time42.
Doctors and nurses have a long and notorious history of being resistant to new technology. While the broad idea of making medicine more efficient, more personalized, and more human would appeal to any doctor, the simple presence of a technology, whether digital medical records or AI diagnostics, doesn’t suggest to them a more creative way to treat their patients. It simply looks like more work for no benefit. Still, I was genuinely astonished at how differently we saw the exact same problem. It was 2012 (for Christ’s sake!), and they had no idea how to integrate novel data into a treatment plan. If you ever say “What the hell am I supposed to do with this data?” over the course of your day, your job is not long for this world.
What should be one of the most creative professions ends up for many being medicine by regression.
In a world of ubiquitous machine learning, patients shouldn’t have to go to their doctor to update a treatment plan. We shouldn’t have humans eye-balling data when machines can make the same judgments and predictions cheaper, faster, and increasingly better. What should be one of the most creative professions ends up for many being medicine by regression43. Neither the spreadsheet full of data I brought to our first visit nor the Jitterbug system I later developed to monitor my son’s diabetes made doctors more creative just by being available. Jitterbug automates the routine treatment planning that takes up about 80% of an endocrinology department’s day. Doctors and nurses could in theory be more productive if that automation freed them to invest in more personalized and creative treatments for individual patients. Unfortunately, they have neither the day-to-day inclination nor the long-term training that would prepare them to leverage automation and move beyond routine labor.
I know that many may look at recruiting, a job that requires no formal training, as a largely routine, non-creative job. We may not want to admit it, but many of us certainly see teachers in that same light. But doctors44? These are among the most elite, highly-trained professionals most of us ever interact with. If AI is meant to augment us all, if it is a complementary technology that increases all our marginal productivities, then surely doctors must be at the forefront of that augmentation. If only doctors had studied economics instead of medicine, they’d know that they were doing it all wrong.
JiffyLube Colonoscopy
Some very big companies are devoting a great deal of marketing and sales to artificial intelligence. They talk about building AI for wonderful ends: “Our systems will make everyone better. Imagine that your doctor is now a superdoctor. With our help, they’ve been collecting data from your watch and your home and your smart toilet45. We’re giving your doctor the time to think of you as a unique patient...no, unique person! And when you do come in for a visit, right there in the office, they’re using our smart sonogram and its integrated behavioral diagnostics to find that tiny little brain tumor, no referral needed!”
I call this the AI bait-and-switch. Their bait is true. It’s real. AI truly can be used to augment doctors and other professionals for the better. I believe in the possibility of this story so much that I spend my days inventing that very bait. The intention of Jitterbug, as well as Socos Labs’ work in Bipolar disorder, autism, and traumatic brain injury are all about making people better, not just their medical outcomes, but their life-outcomes. The bait is a realizable future, though one with an enormous amount of work yet to be done. But as with any good bait-and-switch, what they’re selling isn’t what we’re buying. The bait is superdoctors; the switch is the JiffyLube Colonoscopy.
When I was a kid, I watched my father, a gastroenterologist, scope a lot of people46. He would work the controllers of the endoscope with a finesse only dreamed of by E-Sport professionals. His body would twist and contort in that magical dance of topspin familiar to the gallery watching Tiger Woods will the ball into the hole. He’d say, “See that duct right there?” and before I could say, “I don’t see shit, dad,” there’d be a whole new image on the screen. He’d grab growths, benign and not, that were completely invisible to my untrained eyes. And his patients loved him47.
In the JiffyLube Colonoscopy, instead of seeing that gastroenterologist, you visit an automated clinic staffed by lab technicians. Like the team of hardworking high school grads staffing the local oil-change shop, the clinic’s staff aren’t highly trained professionals. They have no medical degrees, and perhaps not even university degrees. By the virtue of AI augmentation, however, they’re able to execute much of the routine labor of traditional doctors. Automation grants my father’s eye to anyone, without the decade of medical training, or the subsequent decades of increasing skill and experience. Instead of becoming one with the endoscope, the technician stares at an annotated screen, marking in fluorescent green all of the invisible polyps no untrained eye could ever see. The tech is in the room to guide the process, such as making certain there is actually a patient on the table and getting the scope into place. Together, the AI-augmented technician navigates the distal tip close to one of those green, glowing blobs and then they hit the biopsy button. The system autopilots the excision and immediately begins the diagnostics. The patient walks away with a set of standardized treatment recommendations in their app.
The economic reality is not that everyone’s marginal productivity is increased, but rather that the jobs demanded by these new workflows are transformed to maximize productivity.
Yes, machine learning could turn my father into a superdoctor and slightly improve his marginal productivity. Possibilities aside, AI is primarily being used to deprofessionalize. An increasing number of medical jobs that require highly-trained professionals can now be filled by these mid-level technicians augmented by AI. By automating highly valuable medical decisions–setting insulin pump parameters, conducting colonoscopies, reading sonograms, and predicting manic episodes–AI lowers the skill threshold for these jobs and expands the potential workforce that can fill them. The economic reality is not that everyone’s marginal productivity is increased, but rather that the jobs demanded by these new workflows are transformed to maximize productivity. While those transformed jobs might include a small number of “superdoctors”, the productivity sweet spot is deprofessionalized labor. All the things society promised would protect you from automation–university degrees, elite skills, being male48–are no longer the guarantee to a rich career.
It turns out a lot of what doctors do is non-non anyway. A couple of hours in their day is genuinely creative labor, but most are not. People walk into their offices presenting the same sets of symptoms for the same common disorders, all of which have standard treatments. Jerks like me can build AIs to handle the bulk of this routine labor, often for a fraction of the price and in a fraction of the time. Who wants to play Russian Roulette with surprise hospital pricing when a $10/month app subscription might someday cover most of your healthcare needs. Got a weird mole? Snap a photo. Family history of Parkinson’s? Google’s watching your web. Feeling gassy? Consult Janet, your super toilet49. All of this makes medicine more accessible. Who wants to die of a diagnosable cancer just to keep a doctor in the loop?
Elder Care as Vengeance
I was once on stage at the Milken Institute talking about the future of work when one of the other panelists brought up the well-worn idea of retraining everyone displaced by AIs to work in elder care. This idea is motivated by a misunderstanding of the term “social skills”. Its advocates think, erroneously50, that because “social skills are important and can’t be automated by machines” we should train the leftover workforce into “social” jobs like elder care.
Elder care is a go-to example partially because, from a skills perspective, everyone can do it. Of course if everyone can do it, we’re just talking about another non-non job with limited autonomy or wage power. When I said this on stage, my fellow panelist went ballistic on me for “lowering” a noble profession, to which I could only wonder, “Is this your dream job for your own kids?” I certainly agree that caring for the elderly should be a noble profession, but if we truly cared about them, then elder care workers wouldn’t be working multiple jobs at multiple facilities just to pay their rent51.
We’ve already noted our research on the powerful and creative role that social skills play in all jobs. Those elder care advocates mistake people’s complex social skills for jobs in which you interact with people. One is a defining characteristic of what it means to be human; the other is working the returns desk at Target. Besides, advocating for dumping the otherwise unemployable on our aging parents is hardly a morally justifiable position in any case52.
JiffyLube Economy
“Our field isn’t quite ‘artificial intelligence’–it’s ‘cognitive automation’: the encoding and operationalization of human-generated abstractions/behaviors/skills.”
– François Chollet
The automation embodied in textile mills displaced enormous numbers of skilled, artisan weavers. It did so not by automating the cognitive aspects of weaving, like design, but rather the physical process of turning wool into bolts of cloth. Historically, automation has lifted productivity by operationalizing the physical skills and expertise of highly trained individuals. In fact, deprofessionalization of artisan weavers was already in full swing long before the first colonoscopy ever took place. As noted by historian Carl Benedikt Frey, “Early factory machines, it is true, were simple enough to be operated by young boys. And as a result, middle-income artisan craftsmen were replaced by children working for a fraction of their wages in the factories53.” Frey continues that by the “1830s, children workers comprised half the workforce employed in textiles.” Replacing highly skilled artisans with unskilled child labor is the definition of deprofessionalization.
While automation-induced deprofessionalization has been present since the earliest days of the industrial revolution, artificial intelligence adds a profound new dimension to the story by going beyond the physical. As Chollet’s quotation above suggests, it is cognitive automation that will now transform our working lives. In recent years, we’ve seen machine learning match human professionals in an increasing number of sophisticated cognitive skills and knowledges throughout our economy.
We’re not just looking at the JiffyLube Colonoscopy. Routine cognitive labor dominates the work hours of huge numbers of programmers, analysts, accountants, lawyers, and many other professionals. The efficiencies of AI will favor the same deprofessionalization in these fields as in medicine and weaving. We might find ourselves living in a JiffyLube Economy.
In 2017, a little competition was run at Columbia University between some human lawyers and a program developed by the startup LawGeex. They had built an AI to find all the flaws and loopholes hiding in boilerplate contracts. In this competition, both sides read through a collection of non-disclosure agreements and had to flag all of the crucial flaws that would cause the signers nightmares in the future. At the end of the day, the AI had found 94% of the loopholes while the humans had only found 85%. Close enough...I mean, they’re only human. So let’s call it a tie.
The real story was a matter of time. On average the human lawyers took 92 minutes to read each contract54. The AI took 26 seconds. LawGeex is far from the only company looking to automate parts of the process of law, but they are crystal clear about their role in the bait-and-switch. “If a computer can accomplish the repetitive, soul-sucking parts of many jobs, that will free up human talent to work on more complex and creative problems.” Hypothetical superdoctor, meet hypothetical superlawyer. AI-enabled lab tech, meet your new deprofessionalized flatmate, AI-enabled paralegal.
I doubt anyone is surprised that when my wife and I sent our massive spreadsheet of data to our son’s endocrinologist, they had no idea what to do with it. There is, however, an entire category of jobs that spends every day staring at spreadsheets (or their grown up alternatives like Bloomberg Terminals). Analysts live and breathe reams of data to model risk, identify investments, or approve loans. You might think this relative sophistication with numbers would make them less vulnerable to deprofessionalization than those data-phobic doctors, but the simple truth is that no human can eyeball the quantity of text and numbers flowing through financial markets. A growing number of tools, such as JP Morgan’s COiN program, can complete hundreds of thousands of hours of analysis in seconds. This astounding performance difference leads to the inevitable bait-and-switch, “People always talk about this stuff as displacement. I talk about it as freeing people to work on higher-value things, which is why it’s such a terrific opportunity for the firm.” It’s true, the speed of AI analysts will surely create a specialized class of superanalysts that know what to do with the output of systems like COiN. Yet the vast majority of this highly routine labor will either be deprofessionalized or even fully automated.
And what about programming, that job of the future, that skill every young child in America must master? Just as with every other profession, there are large numbers of startups, research programs, and scientific projects looking to apply AI to automate away the boring stuff. Some spit out coding instructions in response to natural language queries (if you’re not a computational linguist, this means you just ask it a question and it tells you how to do it). Others actually write entire programs on their own, cleverly blending machine learning and old-school AI to take care of all the boilerplate code that sucks up so much of a developer’s day.
...if the vast majority of code isn’t written by a machine in ten years, I’ll be stunned.
Today, most AI-powered developer tools truly are about productivity enhancement–essentially sophisticated autocorrect for coders. However, it is the dream of every product designer to be able to talk directly to their computer about the app they’re imagining and have it write all the code to 55. I’ve seen the demos of people working to realize this vision, and if the vast majority of code isn’t written by a machine in ten years, I’ll be stunned56.
Speaking of writing, I am told that “within five years we’ll have scripts written by AI that you would think are better than human writing.” I didn’t even know there was a competition, but apparently the bait-and-switch is too subtle for Hollywood.
Historically, these professions and many others have required extensive education and experience, and, in the absence of cognitive automation, those barriers to entry have given the well-credentialed enormous wage power. The emergence of practical AI, however, has begun to reveal just how much of these expensive professionals’ work hours are routine non-non labor. Very few will want to pay elite salaries for what a computer can do in milliseconds. If you truly believe that you are unique, welcome to every extinct profession you’ve never heard of that came before you.
At its core, the bait-and-switch isn’t a scam; it’s a choice. This is a rational economic choice that improves the accessibility of medical care, legal services, and financial advice. And for all those who believe that AI will create new jobs (and I’m one of them), that’s exactly what these deprofessionalized jobs are: new roles in the economy for people that would never have been able to fill them before. But those new roles are not the amazing creative jobs that many envision; they are in fact even more non-non, with low autonomy, low wage power, and minimal economic mobility.
Some envision an AI utopia in which we are all the equivalent of superlawyers. Others see an inescapable logic of economic equations delivering productivity gains for all. Both of these visions assume that AI is like bionics: slap it on Steve Austin and he runs faster and approves more loans57. But most automation doesn’t directly augment individuals; it transforms the entire system, producing entirely new jobs. Rather than lazily assume that all of those jobs will be superprofessions or that all labor will see productivity gains, we should instead explicitly answer the questions: what jobs will maximize total productivity, and who is qualified to fill them?
Deprofessionalization or Complementarity
“AI will increase everyone’s marginal productivity, making everyone more valuable, not just elite workers. And so AI will inevitably lead to more, better jobs for everyone. Across all of the last 200 years of human history employment has always risen, even at the worst moments.” ”
– Co-Panelist generously demonstrating the Dunning–Kruger effect for the audience58
First, that last is untrue on many levels.
Second, their argument rests on the mistaken assumption that automation boosted people's productivity directly59 and that “bionic” augmentation affects everyone equally. Instead, most automation, including AI, increases productivity by changing work and creating new jobs that are, by virtue of the new system, more productive. Historically, most of those new jobs have been within the scope of capability of nearly all working populations. But there is no guarantee that this will hold true for all automation-driven labor shifts, and absolutely with the facile pseudo-mathematical certainty of the above claim.
In contrast to this claim, we can look at extensive theoretical and empirical work by the two noted MIT economists, Daron Acemoglu60and David Autor61. Amongst their many papers on this subject, one in particular “What Does Human Capital Do? A Review of Goldin and Katz’s The Race between Education and Technology” looks at the relative returns to both wages and jobs of automation over the last few decades. They show that “factor augmenting technology improvements”, the degree to which a new technology boosts productivity, differs by skill level. Rather than one-size-fits-all productivity boosts, emerging technologies most directly compliment high-skill labor.
As automation of physical labor decreases the demand for lower-skilled routine physical jobs, we might expect a shift to favor higher-skilled (higher paying) jobs over lower-skilled. Acemoglu & Autor show just this in Figure 5 of their paper (re-engineered below as Fig 1). During the 80s (black line) the total labor share shifted in favor of higher-skilled jobs requiring university education at the expense of the very low end. But they also show a completely different effect as a result of the emergence of cognitive automation (personal computers, internet, and more) since 1990. The green line shows the “simultaneous growth of employment in high skill, high wage occupations and low skill, low wage occupations.” This is completely contrary to the idea that AI’s productivity gains will lift everyone.
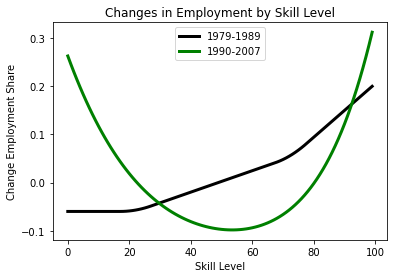
I have been saying for many years that the professional middle-class is about to get blindsighted. It turns out that this has already been going on for three decades. Though we may not think of databases and search algorithms as “artificial intelligence”, their early contribution to cognitive automation has already been hollowing out the middle for 30 years. When I was an undergrad, a professor of mine would routinely send me to the library to collect papers for him. If he went himself, the staff only saw a red-eyed stoner with pizza stains on his t-shirt62 and routinely hassled him before allowing him to leave with materials. So, he sent me, his teaching assistant, in his place to collect all of the books and papers he wanted to read up on. When was the last time you’ve even been in a library, much less sent an underling to look something up for you? (Unsurprisingly, librarians are a 50th percentile job, exactly where demand has dropped the most.)
Acemoglu, Autor, and their colleagues have gone on to model the differential effects of technology by skill level and education, greatly enhancing our understanding of how new technologies affect labor markets. I, however, in my great arrogance, see a shortcoming in their model conflating routine skills with the human factors that drive the creativity economy. Routine skills, however cognitively complex, are relatively easy to automate. This drives deprofessionalization, as lower-skilled labor augmented by AI can be substituted for higher-skilled, just as we’ve discussed in the JiffyLube Economy, leading to low wage dispersion and growth of routine vs non-routine labor. This shifts demand for jobs to the left on the skills graph as indicated by the blue line in the Fig 2. Even demand for math-intensive jobs has decreased since 1980 if those jobs are highly routine and lack social interaction. Middle-skill jobs that once required extensive job training or basic university degrees can now be done by relatively lower-skilled employees, who are more abundant and require less training. We all get the same productivity for a lower cost. This is the core of deprofessionalization, and if it were the only effect at play, we might expect to see a greater boost in employment share at the low end rather than the high.
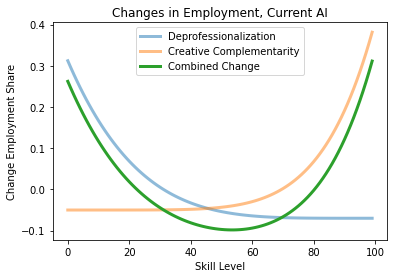
Countering the deprofessionalization phenomenon for routine skills is AI’s unique complementarity to creative labor, superlinearly augmenting its productivity. This is represented in the steep upwards slope of the orange curve in Fig 2. High-skill labor does not necessarily mean high creativity; however, in most labor markets these two are loosely correlated. For simplicity's sake, the orange curve assumes that higher-skill jobs as represented by Acemoglu & Autor also demand greater autonomy and innovation. In fact, existing research suggests that demand for jobs that integrate elite technical knowledge with social skills drives much of the rightward lift. If we look at jobs around the 95th percentile, for example, we see a list dominated by sophisticated managerial and engineering roles.The most innovative practitioners of those jobs will be the most augmented by AI as their routine aspects are automated63.
These two factors, deprofessionalization and creative complementarity, interact to simultaneously produce the boost in employment share for both high-skill and low-skill jobs (the green curves in Figures 1 and 2). Deprofessionalization shifts employment in the middle down towards lower-skill workers, while creative complementarity drives up the productivity of the elite. For example, relatively high-skill labor with modest degrees of autonomy (80th percentile “American dream” jobs: credit analysts, nuclear technicians, financial specialists) have held their share of employment up through 2007. As different industries increasingly integrate artificial intelligence, the demand for creative labor shifts increasingly to the high end. Those 80th percentile jobs like chemists or microbiologists are being replaced by 60th percentile jobs like lab technicians. Only those able to reap the complementary boost from AI can supply the increased demands for the top decile of jobs. This basic effect can be seen in the shifts of the orange and red curves in the Fig 3.
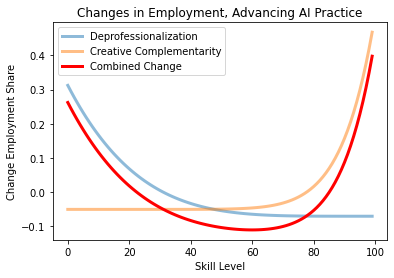
In Fig 4 overlays the empirical change in employment share from Acemoglu & Autor (green curve) with the model estimate assuming an increasing premium for creative labor (red curve). It shows creeping deprofessionalization as AI substitutes for increasingly complex skills. Reskilling is clearly not a solution to this problem as no reskilling program will ever drive an employee up towards jobs that will see increased demand; you can’t reskill someone to become a physicist or a petroleum engineer in six weeks. University education, short of the most elite programs, also does not shift enough labor into the region of increased demand to produce a boost in their employment or wages, and there’s no guarantee that any boost that they see today will be preserved as AI substitutes for ever-more sophisticated routine skills. Can we keep the promise to students that if they invest years of their lives and enormous sums of money to become analysts and nuclear technicians that anything remotely like those jobs will be available to them when they enter the job market?
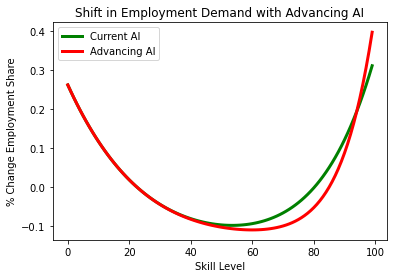
Here again is Table 1, a selection of jobs at each skill-wage level from the Bureau of Labor Statistics. As the chasm between high and low grows (Fig 4), what happens to the people currently working in these four clusters?
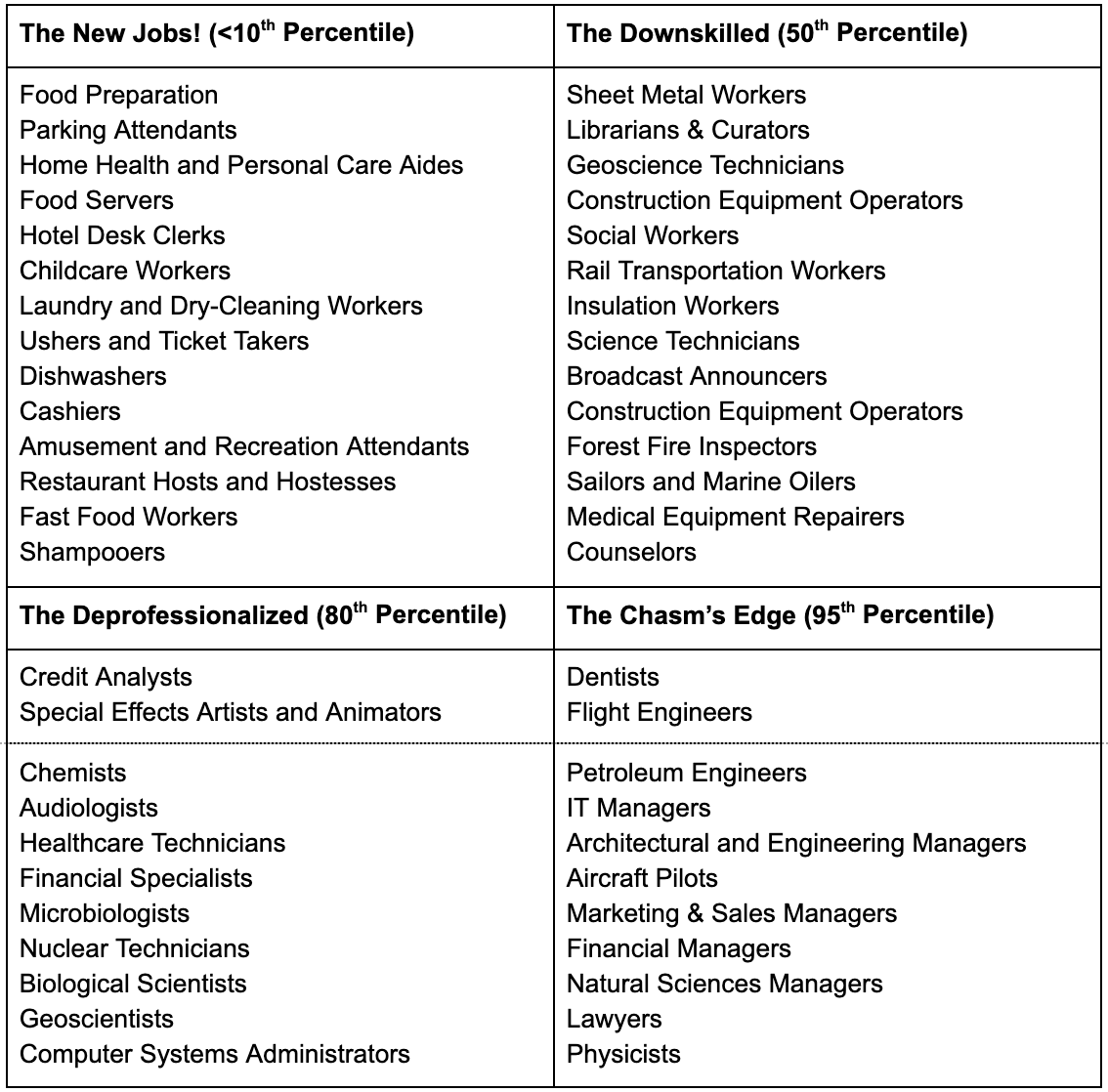
The New Jobs! (<10th Percentile)
When people say, “AI will create more jobs than it destroys”, they mean Starship Captain and Psychic Therapist. Unfortunately, they’re actually referring to these jobs. This group is a mix of routine and non-routine physical and unskilled labor–think farmworkers, food service, eldercare workers. Here we see the effects of AI and automation as entirely substitutive; any task AI performs, it takes away from a person. Barring global labor shortages, the only available response for that person is to do the same task for less. This will result in massive downward wage pressure. One day autonomous robotic automation will become so cheap that it won’t make sense to employ humans for these lowest-skilled jobs, but having a strawberry-picking robot does not increase demand for strawberries, and if I have cheap human labor doing strawberry-picking, I don’t actually need the robot… for now64.
These jobs will proliferate as AI deprofessionalizes the middle class. By sheer global numbers, there is a vast population of potential creatives hidden in these jobs, but they spend every day taking care of your toddler and many nights feeding your grandmother. Even when those jobs are done, there is the choice between rent and food. There are no cognitive reserves to launch a startup or invent a better networking app.
The Downskilled (50th Percentile)
In truth, the Downskilled include a range of jobs in today’s labor market, perhaps from the 30th-70th percentiles. These jobs have typically required extensive training and time at university. The labor is non-non but still skilled. As revealed in Acemoglu & Autor’s analysis, Downskilled jobs already experience a great deal of pressure as the innovation economy expands, and people in these roles are consistently told to upskill or lose their jobs. For the moment, these warnings are fair.
It turns out that the first economic sweet spot in bringing machines and people together is taking the marginally unqualified and making them just qualified enough65. They have lower wage power and education than traditional “professionals”, but augmented by AI they can do many of the same tasks nearly as well. In an Elasticity of Substitution model for skill- and creativity-dependent automation, the equilibrium solution maximizes total factor productivity not by making people better, but by making cheaper people more competitive in the labor market. Any individual in this Downskilled cluster becomes fundamentally substitutable for anyone else because the part of the job that requires elite training is now done by artificial intelligence, and any autonomy has been deprofessionalized away.
With AI’s cognitive automation, Downskilled jobs will include our AI-enabled lab techs and AI-enabled paralegals. But two years later, employees upskilled to use these AI tools will need a whole new set of skills as deprofessionalization inevitably advances. All of their upskilling is treading water, and ever-increasing automation means they may never touch ground again. And to top it all off, they will be treading the same waters as those carried downstream from (Newly) Deprofessionalized jobs.
The Deprofessionalized (80th Percentile)
Speaking of the Deprofessionalized, these jobs are the heart of the AI bait-and-switch. This job cluster requires high-skill, cognitive, but still largely non-non labor. Routine pipetting and spreadsheet modeling fill the days of people filling these jobs. Their parents invested deeply in their education and human capital, and it culminated in university degrees and professional careers as financial specialists, chemists, and geoscientists. AI pitched as “taking care of all the busywork” might seem like a dream to people working in this cluster, until they realize how little is left.
This cluster will be largely deprofessionalized by AI. The required human capital here is expensive, but the routine tasks are ripe for substitution, particularly because so much of these jobs are already digital… no expensive robots! Instead, these Deprofessionalized jobs will shift down to cheaper, lower-skilled, AI-augmented labor66. This leaves the professional middle class with a profound choice. A small percentage will work towards the bait and rise up into the creative economy, but for the most part, those currently working Depreofessionalized jobs will disappear into the chasm. They are the ultimate losers of the switch.
Before you start calling for the heads of those unscrupulous industrialists stealing everyone’s jobs, remember that the gains of automation aren’t just about who gets a job but about sharing the value created with more people through lower costs and greater access. As we already discussed, no one wants to die of a diagnosable cancer just so to keep a human in the loop. And wouldn’t it be great if for the price of an order of McNuggets you could get your McLawyer to review every contract in your life. There are real and immediate benefits to deprofessionalization.
But are cheap sneakers enough67? Is there more to these amazing jobs we’ve been promised than a deprofessionalized service economy? And if there is, will those jobs truly be accessible to all? The “meritocratic” system that evolved around today’s professionals has drawn deep criticism as a mechanism of intergenerational wealth immobility. At the Chasm’s Edge, these cultural barriers to the creative economy may become insurmountable to most.
The Chasm’s Edge (95th Percentile)
As already noted, Acemoglu & Autor’s model contains a problematic conflation68 of skill, wage, and creativity. Now that we’ve reached the jobs on the Chasm’s Edge–Sales Managers, Petroleum Engineers, Pilots–these underlying factors unmistakably diverge. Dentist and Petroleum Engineer all require complex skills and vast knowledge, but how much of the day-to-day of these jobs involves finding novel solutions to uncertain problems? We don’t want Pilots and Flight Engineers “exploring the unknown” with us at 38,000 feet; we want people that will get the damn plane from here to Tahiti without a bump. In contrast, “Manager” doesn’t require the same complex skills 69 as Physicist or Engineer—median wage and MBAs be damned—but creativity in a high-level manager is invaluable.
What defines these jobs is not their skill level but the people inhabiting them. Almost anyone can manage a project (poorly), but the most elite managers are drawn to the projects with the greatest economic value. Armies of engineers and doctors are turned out every year, all with the same skills and knowledges, but the economic value of subspecialties like anesthesiology and petroleum engineering draw the most elite performers into their ranks. It’s not the skills, it’s the people.
...deprofessionalization is a receding tide: what it leaves behind is a complex, unexplored seashore that requires creativity to traverse.
AI’s impact on the Chasm’s Edge is also all about the people. Some of the jobs at the Chasm’s Edge will be deprofessionalized, but we don’t necessarily expect the people filling them now to move down. Is your job uniquely you, or are you filling a role that anyone else can fill? If I can substitute anybody in that role, I can (eventually) substitute an AI and a lower-skilled worker. The only reason job demand shifts to the right in Figures 1-4 is because deprofessionalization is a receding tide: what it leaves behind is a complex, unexplored seashore that requires creativity to traverse. Every task, from every job, that requires that complex exploration shifts to the right into the creative economy. This is the second sweet spot of AI augmentation: creative complementarity emerges from the combination of creativity, domain expertise, and machine learning tools70.
Creative complementarity, not raw automation, drives AI’s greatest value creation. Tapping its full potential demands a society of inventors, whether we invent technology, art, or science. It turns out the personal qualities Gild found to predict job performance, like general cognitive ability, are also the biggest predictors of becoming an inventor, morso than parental income or education. And becoming an inventor is transformative, creating wealth, increasing intergenerational economic mobility, and even lifting the wellbeing of coworkers. Interestingly, those same personal qualities are relatively weak predictors of who becomes a doctor or a lawyer. For those jobs, parental education is the biggest predictor. There is a difference between being educated and inculturated into high-skill jobs and being creative71. Doctors discovering new treatments are obviously inventors, and so are Lawyers arguing a novel legal principle before the Supreme Court. Filling out divorce filings via template… not so much.
Augmented by AI, many people holding jobs from across all of these clusters have the chance to become inventors. Can you imagine the roles of museum curators, forest fire managers, and even elder care workers on the edge of the creative chasm? I may be disdainful of the idea that elder care is the employment solution to all of our automation woes, but against all economic reason, some amazing and creative people already occupy these jobs despite often needing two of them just to pay rent. Every one of these jobs can be reconceptualized as either deprofessionalized service roles or creative endeavors.
The idea that everyone will spontaneously join the creative economy simply because that is where demand is increasing is absurd and completely misses humanity’s repeated (and often gleeful) violation of economic theory. AI will create innumerable opportunities for individuals that have already developed a creative voice, but few others will have the means of rising to fill that demand. This isn’t the Industrial Revolution with farm labor transitioning laterally towards more productive factory labor. “Dry-Cleaning Workers” are rarely becoming “Construction Equipment Operators”, and few “Insulation Workers” are upskilling into “Microbiologists”. Are any of them prepared to become “Architectural and Engineering Managers” just because the demand for those jobs increases? Much less those jobs that are yet to be created? It’s much more likely that there we’ll an expansion of the ranks of “Personal Care Aides”, earning less than a living wage (and contracting Covid-19 in the process). We can dream about what “AI Ethicist” and “Elder Care Worker” could be in some Rodenbergian future, but today they’re misery.
For my part, I see two possibilities. In the first, we thoughtlessly allow cognitive automation to greatly accelerate the expanding rift that has always existed between creative labor and the rest of society. In the second, we choose to build explicit systems for bridging that chasm, creating an infrastructure of economic mobility. There are other choices beyond and within these two possibilities, including having no AI for anyone, but the real scam of the AI bait-and-switch is pretending there is no choice at all–that one future or another is inevitable.
If we believe in the lazy myth, we’ll get the switch. If we put in an effort to grow ourselves, we might still get the bait.
The collected works of Prof. Schlashenschlessen
“We don’t even need universities anymore; we can just send them all to trade school to get upskilled, just like in post-war Germany.”
– Pompous Mansplainer
History’s most famous reskilling effort is the Titanic transition of naval workers to the automotive industry in post-World War II Germany. That grand economic retooling was, in some respects, revolutionary in its success. But that very success now fuels the willfully naive assumption that we should adhere to this Teutonic template and reskill our modern workforce into new jobs as well. I reveal for the first time a newly discovered workplace memo from one Bubba Daimler–fascinatingly, the grandson of one of the company founders72–who supervised just such an operation.
The transition of skilled laborers from ship to auto is singular for its specificity and is often hailed as a monument to the success of reskilling, but take note that many jobs described in this artifact, those modular enough for retraining, have since been lost. Covetable jobs that have been retained and remain vital today are predominantly those that sneer at simple retraining: high-skill workers and high-value managers. As such, the achievements of Herr. Bubba73 and his contemporaries must be understood with some nuance as to what “successful” retraining actually implies.
From the Daimler-Benz Corporate Archives: August 17, 1956
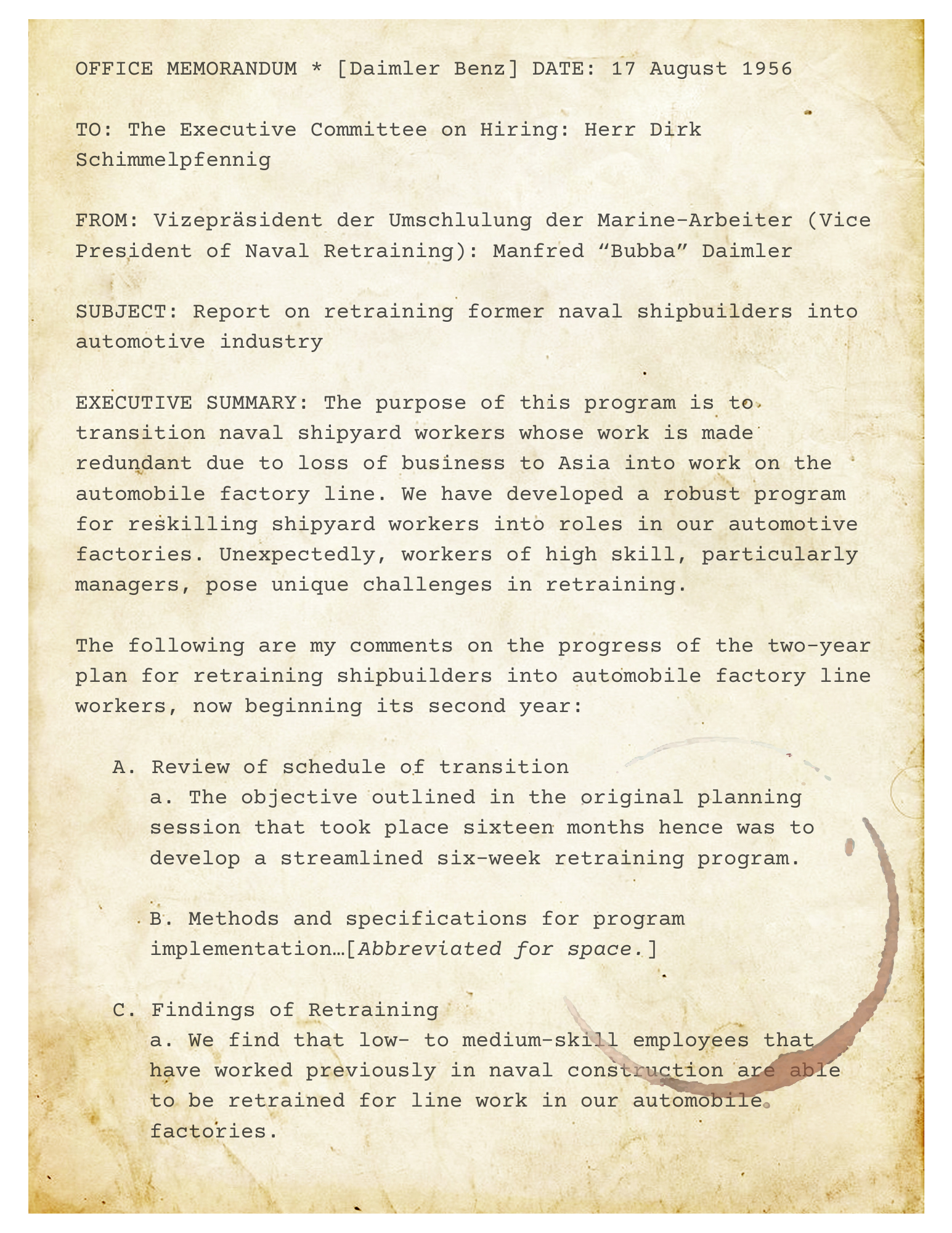


...says the vastly wealthy grandson of the visionary who founded the company. After pouring over the company records, there is no doubt in my mind that Bubba Daimler is highly qualified for his role. Daimler, Benz, Maybeck, and numerous spontaneous creatives of their time hoisted their companies into greatness out of naught, but followed through by investing heavily in their offspring. They ensured their legacy not just through the transmission of wealth but also through the intentional bolstering of the human capital of their beloved children. Given the rather unexceptional career of Bubba’s lackadaisical brother, it’s clear that his familial connection may not have necessarily entitled him to a prominent role in the company, but it did prepare him for it. The rise of the automotive economy brought with it an explosion of creative jobs–designers, engineers, and marketers–but Bubba and his peers are representative of a truly inherited creative class that was trained to occupy many of these positions from the day they were born.
The transition of skilled laborers from ship to auto is singular for its specificity and is often hailed as a monument to the success of reskilling, but take note that many jobs described in this artifact, those modular enough for retraining, have since been lost. Covetable jobs that have been retained and remain vital today are predominantly those that sneer at simple retraining: high-skill workers and high-value managers. As such, the achievements of Herr. Bubba and his contemporaries must be understood with some nuance as to what “successful” retraining actually implies.
“Reskilling” may be shouted self-servingly as the future of work, but it becomes evident over time that simply reskilling people into different jobs will not improve their long-term prospects because their intellectual experiences have not fundamentally changed74. It is also vital to acknowledge that during Bubba’s time, companies like Daimler developed programs with truly minimal retraining periods. Bubba’s final note that it is “just too late” for these workers assumes that any complex job could have been retrained in a mere six weeks to begin with75!
Post-war social safety net programs, investments in human capital, and a reshuffling of wealth from the Gilded Age did result in higher paying jobs for the middle-class. Though workers and unions were undeniably robust during this ravaged period, wage-power did not lead the expansion of the creative economy; they were just listening to the same music. Rather, the rise of the middle-class in the 1950s was due to a reinvestment of industry returns into the service economy. At this time, those in the service economy were of relatively high-skill, and the middle-class was still a viable pathway towards entering the creative economy for those excluded from the “cultural transmission” that privileged Bubba and his colleagues. It was still a time when hard work could conceivably pay off.
The memo closes with Bubba’s acknowledgment of the company's desperation for creative talent, a phenomenon we still see in global industries today. They may have been hungry for routine labor on the factory floor, but that hunger was matched if not superseded by a thirst for creative talent76.